Businesses today rely on the world of big data for resources. Major corporations have become the primary sources of information. Google, Facebook, Amazon, Twitter, and other companies are the hotspot for media and products. These social networks, platforms, and websites continuously build, improve, collect, and process users’ profiles and information to suit their products and content.
Business intelligence and augmented analytics have become the go-to factors to consider when using any data source. In recent years, companies have developed new business tools and AI-driven platforms to handle the amount of data required for efficient analytics, marketing, and product placement. New companies will need these platforms to grow from a humble startup.
Data-driven marketing is a pivotal point in entrepreneurship and business as a way to get more control and data points faster than traditional marketing methods.
This article will discuss how business intelligence and augmented analytics fuel and enhance current data-driven marketing endeavors. Also, we’ll discuss whether they will become indispensable for new companies looking to carve a user base.
The Importance of Data and Analytics
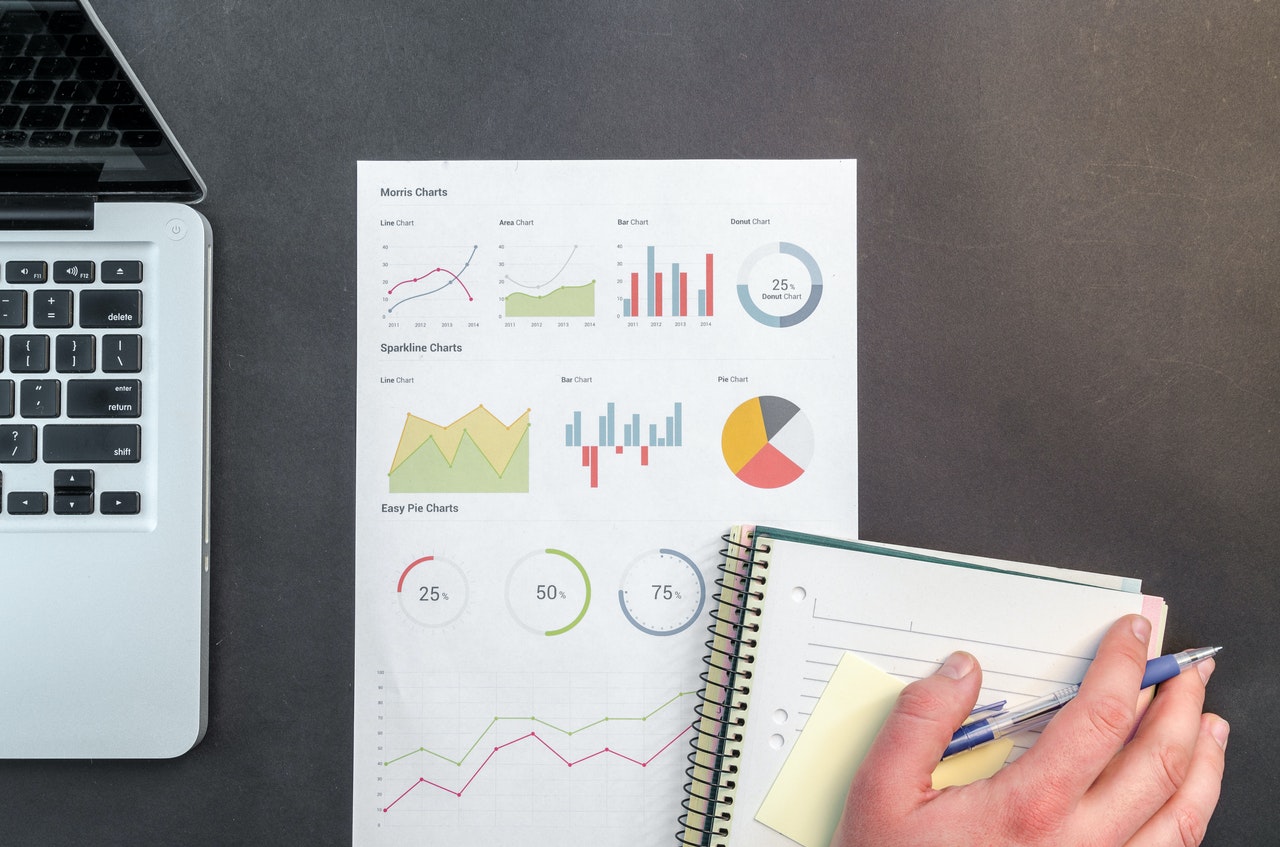
Organizations often rely on considerable amounts of data to remain competitive or serve the entire population. Companies like banking, healthcare, government, retail, and manufacturing need to make quick decisions in line with current trends and predict future causes and effects to stay ahead of any issues. Data analytics helps expedite the decision-making process and company growth.
Analytics provides two-fold benefits:
- Focusing existing insights, effects, and outcomes into data that businesses can use, trade, or sell
- Developing current data into models to predict decision causality and improve the decision-making processes and velocity
By using big data analytics, companies can develop a competitive edge over traditional methods and get the most up-to-date inputs for their marketing and development decisions and efforts. If used correctly, data analytics will achieve:
- Faster decision-making and more targeted marketing campaigns
- Improved campaign follow-through and actionable content
- Data-based products and solutions
Analyzing data is a never-ending, self-feeding process. Processing existing data points will provide additional information your company can leverage or sell on the market to grow and fuel other analytics.
Business Intelligence and Tradition
Business intelligence has become commonplace jargon often used as a buzzword in the marketing world. The term effectively gives a new scale and a way to organize and achieve company success. It defines how modern enterprises are heralded through patterns, data, and a statistics-based approach. Business intelligence relies on statistical analysis, predictive models, and human management to get the highest company value.
Business intelligence (BI) tools are the primary way a new business can achieve a meaningful level of intelligent decision-making and statistical analysis to operate and compete. These tools help organizations compile, show, organize, and process past data with crisp data visualization tools, graphs, and modes. BI tools often require a team of data analysts who can leverage the existing raw data points into new insights.
Overall, BI tools help companies achieve the following:
- Better and faster ways to generate revenue on the current market
- Provide accurate market insights from previous examples
- Get information on competitor products and services
- Increase customer satisfaction and retention
- Track company and department performance
- Detect process inefficiencies and redundancies
- Streamline the decision-making process
Current BI Tools to Use and Explore
Once data is in the appropriate storage, BI tools extract it and analyze it to benefit the client. Here are some of the most popular BI tools on the current market.
SAP Business Intelligence
With SAP, companies get real-time insights and intelligence evaluations. The tool primarily uses a self-serve method to expand BI, predictive analysis, and encourage product and service planning. SAP is widely recognized for its cloud support and scalability.
SAS
SAS is an excellent tool to report current events and provide a streamlined approach for predictions and data analysis. Its high-level data integrations make it a compelling choice for analysts, statisticians, and business forecasters.
Tableau
This BI tool emphasizes user-friendliness. It’s compatible with the most major platforms to deliver and process data, including MS Office Suite, Oracle, and Google Analytics. Tableau connects to databases and provides streamlined visualization to expedite decision-making and data analysis.
Sisense
Sisense brings data from within the company and pools it with external information to provide an accurate outlook on the current impact. It focuses more on allowing non-technical employees to comb through large databases quickly.
QlikSense
This new-generation BI tool creates data-bound relationships and helps visualize the impact these data points have on one another. It creates new extrapolations points that aren’t accessible through regular DB queries.
MicroStrategy
This dashboarding BI tool monitors trends and provides a quick overview of larger data points to recognize potential growth opportunities. It collects data from various sources and can integrate with corporate and cloud software on demand.
Microsoft Power BI
This web-based analytics suite excels in showcasing data visualization capabilities and forming real-time overviews. Since it’s web-based, it’s an excellent solution for teams that don’t work in a single office or travel frequently. Microsoft offers seamless integration with other apps to bolster reports and provide more valuable insight.
The BI Tool Demands and Implementation Challenges
BI tools are indispensable AI-driven services that help companies in daily operations. However, all BI tools are just a blunt instrument to showcase information in a presentable manner. Data scientists are doing all the work from then on, combing and combining that data into meaningful reports.
Data scientists can become the most valuable members of a marketing team. They can use currently available user data to create a model to interpret the information. Data scientists can pool past purchases, consumption patterns, and user behavior into a predictive model to generalize the behavior. This generalization can become the backbone of a successful marketing campaign to grow the company.
One major problem with data scientists is that they are crazy expensive. According to Glassdoor, their base median salary starts at six figures and can often only go up from there. A startup or a smaller company generally can’t afford their services in the long term.
You can automate some of the data analysis processes to reduce costs and deliver similar results. This is where augmented analytics shines.
What Is Augmented Analytics?
In the simplest terms, augmented analytics combines AI and BI. Augmented analytics uses machine learning to automate some of the vital processes data scientists provide to supplement human resources and improve research.
When appropriately used, augmented analytics offshores some of the tasks usually relegated to BI tools and data analysts. Modern tools allow for data preparation, analysis, insights, and data visualizations. Using a single tool to achieve these tasks saves money compared to hiring a data scientist and implementing a dedicated BI tool to work in.
Benefits of Augmented Analytics
The primary benefits of using augmented analytics are combining machine learning to supplement (and replace) some previously human-only resources and BI tools. These include:
- Allowing a more accessible data entry point
- Using BI tools without human input
- Processing raw data into insights
- Timely and accurate information and reports
- Quicker data processing
- Reduced human dependency
- Reduced workload requirements
- Lower overall costs compared to BI tool + data scientist salary
- Higher accuracy and less chance for human errors
BI Tools vs. Augmented Analytics
BI tools most often provide raw data in a more visual form with additional connections that make that data easier to manage and interpret. Because these tools don’t process data to provide insights, companies still have to rely on human data scientists to do the heavy lifting.
On the other hand, augmented analytics automates data transformation using machine learning. These services require little to no human supervision and all but remove the need for hiring a data analyst. Any department can use the output to further company growth and expedite success.
Some BI tools have caught on to the benefits machine learning offers in compiling insights and have begun implementing augmented analytics into their packages.
Augmented Analytics Going Forward
Augmented analytics is a relatively new field, but AI development has spearheaded its implementation and progress. There are three main steps that augmented analytics currently focuses on:
Data Cleaning and Preparation
Cleaning and processing data for use are some of the most labor-intensive processes that heavily slow down data analysis. Augmented analytics can offload most or even all of the tasks to automated tools. Some BI tools have already begun using AI to clean and prepare data for presentation and processing.
Pattern Detection
The human mind is unparalleled in spotting patterns in data, but AI tools have slowly begun to catch up. While automated tools still require human help to get the process through, they can significantly speed up the pattern detection process.
Actionable Findings
At this stage, augmented analysis needs to record patterns created by data scientists and suggest actions. The system also needs to track current processes and supervise implementation. It needs to offer insights on operational effectiveness and get the company on the right track if there are any errors.
The third stage requires more work and still primarily relies on human resources.
Where Next?
In the past, data scientists were the only way to get more information from past data and resources. Advances in data processing and machine learning are slowly able to offload some part of the process to automated tools.
These advances help smaller businesses achieve more success by cutting down costs and leveraging real-time data from big companies.
Disclosure: We might earn commission from qualifying purchases. The commission help keep the rest of my content free, so thank you!